What Graphics Card Is Best For Ai? Top Picks Revealed
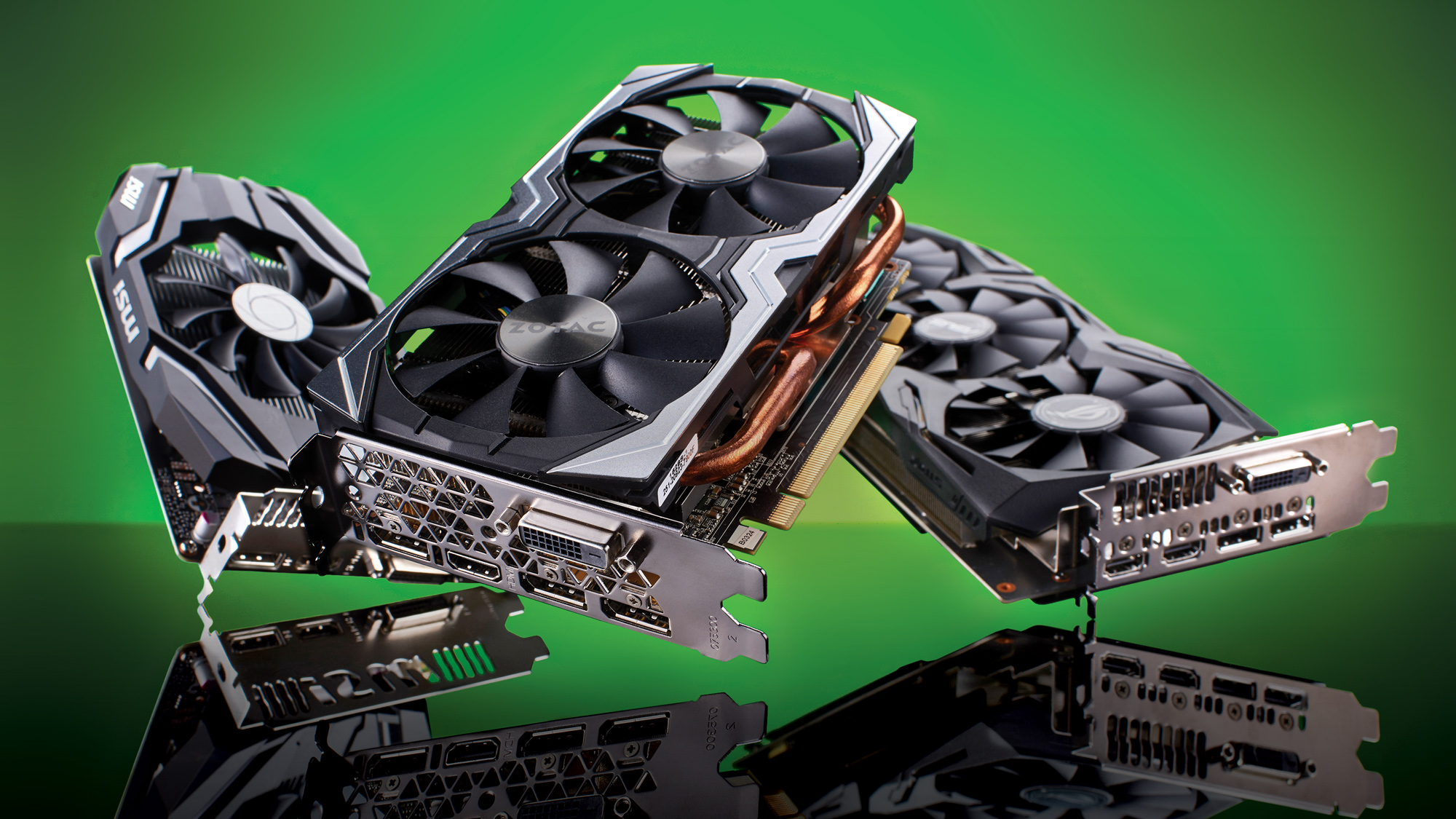
The realm of artificial intelligence (AI) has witnessed unprecedented growth in recent years, with applications spanning from natural language processing to computer vision. A crucial component in unlocking the full potential of AI is the graphics card, which plays a pivotal role in accelerating complex computations. In this article, we will delve into the world of graphics cards tailored for AI, exploring the key considerations and top picks that can elevate your AI endeavors.
Understanding AI Workloads
Before diving into the best graphics cards for AI, it’s essential to understand the nature of AI workloads. AI computations often involve matrix operations, which are inherently parallel and can be efficiently processed by the massively parallel architecture of modern graphics processing units (GPUs). This makes GPUs an ideal choice for accelerating AI tasks compared to traditional central processing units (CPUs).
Key Considerations for AI Graphics Cards
- CUDA Cores/Stream Processors: The number of CUDA cores in NVIDIA GPUs or stream processors in AMD GPUs directly influences the processing power. More cores mean better performance in parallel tasks like AI computations.
- Memory and Memory Bandwidth: AI models, especially those in deep learning, require a significant amount of memory and high memory bandwidth to handle large datasets and complex models efficiently.
- Power Consumption: High-performance GPUs often come with higher power consumption. Ensuring your system can support the power requirements of your chosen GPU is crucial.
- Cooling System: Efficient cooling is vital for maintaining performance during lengthy AI training sessions. Look for GPUs with robust cooling systems.
- Software Support: Ensure that your GPU is supported by popular AI frameworks like TensorFlow, PyTorch, and Caffe.
Top Picks for AI Graphics Cards
1. NVIDIA GeForce RTX 3090
- CUDA Cores: 10,496
- Memory: 24 GB GDDR6X
- Memory Bandwidth: 936 GB/s
- The NVIDIA GeForce RTX 3090 stands out as a powerhouse for AI applications, offering unparalleled performance with its ample CUDA cores and high memory bandwidth. Its support for ray tracing and AI-enhanced graphics makes it a versatile choice for both gaming and professional applications.
2. NVIDIA A100
- CUDA Cores: 6,912
- Memory: 40 GB HBM2
- Memory Bandwidth: 1,555 GB/s
- Although primarily aimed at data centers and professional applications, the NVIDIA A100 offers unmatched performance for AI workloads, especially in areas like natural language processing and computer vision. Its high memory capacity and bandwidth make it ideal for large-scale AI models.
3. AMD Radeon Instinct MI8
- Stream Processors: 4,096
- Memory: 32 GB HBM2
- Memory Bandwidth: 1,024 GB/s
- The AMD Radeon Instinct MI8 is a competitive offering in the AI graphics card market, providing a balance between performance and power efficiency. It’s well-suited for deep learning tasks and offers robust support for AI frameworks.
4. NVIDIA Quadro RTX 8000
- CUDA Cores: 4,608
- Memory: 48 GB GDDR6
- Memory Bandwidth: 672 GB/s
- Designed with professionals in mind, the NVIDIA Quadro RTX 8000 offers high-end performance for graphics-intensive applications, including AI-enhanced workflows. Its large memory and specific optimizations for professional software make it a strong contender.
Conclusion
Choosing the best graphics card for AI depends on your specific needs, including the type of AI applications you’re running, your budget, and your system’s capabilities. NVIDIA’s GeForce and Quadro series often lead in AI performance, but AMD’s offerings provide viable alternatives, especially for those already invested in the AMD ecosystem. Remember, the best GPU for AI will be one that balances performance, power consumption, and cost, tailored to your unique requirements.
FAQ Section
What is the primary factor to consider when selecting a GPU for AI applications?
+The number of CUDA cores or stream processors is a critical factor, as it directly affects the GPU's ability to handle parallel computations inherent in AI tasks.
Can any GPU be used for AI, or are specific models required?
+While technically any GPU can be used for AI, high-performance GPUs with more CUDA cores/stream processors and ample memory are significantly more efficient and effective for complex AI workloads.
How does power consumption impact the choice of a GPU for AI applications?
+High-performance GPUs often require more power. It's crucial to ensure your system's power supply can support the chosen GPU, especially during prolonged AI training sessions.
Final Thoughts
The landscape of AI and graphics cards is rapidly evolving, with new technologies and models emerging regularly. Staying informed about the latest developments and understanding the specific requirements of your AI projects will help you make the most informed decision when selecting a graphics card. Whether you’re a professional developer, a researcher, or an enthusiast, choosing the right GPU can significantly enhance your AI computing experience and productivity.